Authors: Aris Galanopoulos, Senior Product Manager and Iliana Kerzeli, Senior Customer Success Associate
Why is benchmarking crucial in the pharmaceutical industry? Benchmarking, an essential tool in drug development, allows companies to assess the likelihood of a drug making it through clinical development and receiving regulatory approval. While several methods exist to assess a drug’s probability of success (POS), surveys we conducted at several drug portfolio strategy-focused conferences clearly showed that historical analysis/benchmarking is the preferred method for assessing POS.
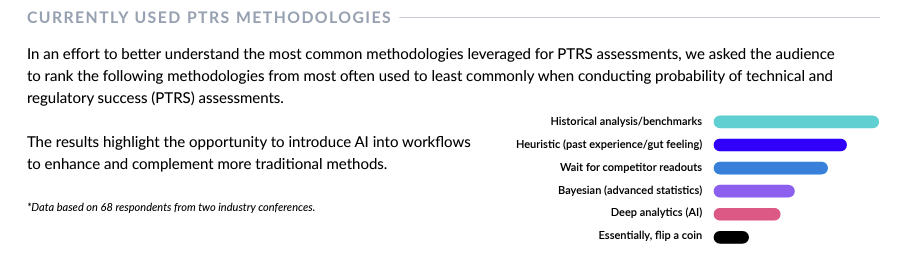
In this blog, we’ll discuss the importance of benchmarking, highlight benchmarking-related challenges faced by pharmaceutical professionals and show how you can level up current static benchmarking approaches to put your decision-making on a more solid foundation.
The Importance of Benchmarking in the Pharmaceutical Industry
Benchmarking involves comparing a drug candidate’s performance against historical data from similar drugs. This process helps identify potential risks, enables informed decision-making and improves the overall efficiency of the drug development process. Understanding the past can help you plan for the future.
Benchmarking is highly relevant for the following applications:
- Risk Management: Drug development is inherently risky, with high rates of failure, especially in the early phases. Benchmarking makes it possible to get a better handle on the likelihood of success at each clinical development phase. Companies can mitigate risks by understanding where and how similar drugs have succeeded or failed.
- Resource Allocation: Benchmarking allows pharmaceutical companies to allocate scarce resources strategically by identifying candidates with the highest potential for success. This ensures that funds, time and efforts are directed toward the most promising drug candidates.
- Decision-Making: Benchmarking provides a data-driven foundation for decision-making. It empowers stakeholders to make informed choices about whether to continue, pivot, or terminate a drug development project based on empirical evidence from past experiences.
- Regulatory Strategy: Regulatory approval is one of the most critical and challenging phases of drug development. Benchmarking helps companies understand regulatory requirements and trends, allowing them to design their trials and documentation to meet regulatory agencies’ expectations.
Traditional Methods of Benchmarking in the Pharmaceutical Industry
Traditionally, several approaches are used to benchmark a drug candidate’s performance. The most relevant one involves analyzing historical data from past drug development projects, including success rates at different stages, timelines and reasons for failures.
Another method is clinical trial simulation, where computational models are used to simulate the outcomes of clinical trials based on existing data. Simulations can predict the likelihood of success, identify potential pitfalls and help optimize trial designs.
In comparative effectiveness research, the effectiveness of a new drug is assessed against existing treatments. This gives companies a better grasp of the competitive landscape and the new drug’s value proposition.
These approaches can yield valuable insights, but they also have significant shortcomings, such as manual and error-prone efforts, limited data access, lack of standardization, and outdated data. These challenges lead to legacy solutions often underestimating the risk associated with drug development and, therefore, providing an overly optimistic take on the probability of success.
In comparing Dynamic Benchmarks to legacy industry solutions, we found up to a 16% overestimation of phase transition success rates and an average of 8% overestimation of overall approval success.*
Addressing Short-Comings of Traditional Benchmarking Approaches
The most critical components required for more accurate benchmarking are better data and improved analysis methodologies. When it comes to analyzing historical data from past drug development projects, common challenges that traditional benchmarking approaches include:
- Data completeness: New drug development data that can inform and refine benchmarking is generated daily. Most existing benchmarking solutions are updated infrequently and, therefore, don’t draw on the most up-to-date information.
- Data availability and quality: Typically, data used for benchmarking is provided at a high level and is often unstructured and not detailed enough to obtain reliable benchmarks. For example, to obtain an accurate benchmark for a drug treating HER2 negative breast cancer, looking broadly at oncology or even breast cancer data is not sufficient. More detailed data sets that provide program-based historical approval rates are needed to understand the different factors that impact the success of a novel drug targeting a specific cancer or cancer subtype.
- Data aggregation: Data aggregation currently has severe limits as it does not consider all relevant information. For example, innovative drug pipelines, e.g., those that skip phases or have dual phases, are often not accounted for.
- Data search and filtering: The ability to sort data by a multitude of dimensions, including advanced filtering options, is essential for the user to make the most accurate assessments. Typical one-size-fits-all approaches to data manipulation and filtering limit the usefulness of the data and do not allow for benchmarking for exact treatment settings.
- Subpar methodology: POS calculations are typically generated by multiplying phase transition success rates. This overly simplistic approach tends to overestimate a drug’s success rate, resulting in less-than-ideal data for decision-making.
Large amounts of harmonized, curated, structured, and current data are needed to level up benchmarking efforts in the pharmaceutical industry and obtain an accurate view of success and risk. Combining those data with improved analysis methodologies that take a more nuanced approach can address the risk of overestimating the POS of drug development programs.
Raising the Bar With Dynamic Benchmarks From Intelligencia AI
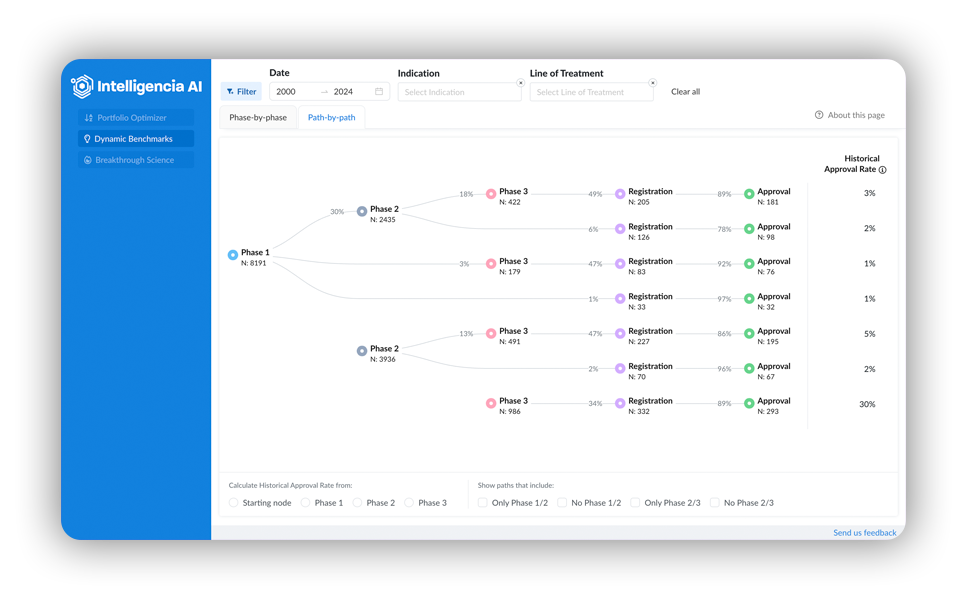
With Dynamic Benchmarks, Intelligencia AI has created a solution that redefines benchmarking in drug development and mitigates the challenges associated with traditional approaches:
- Data Completeness: Data collection and curation pipelines incorporate new data in close to real-time, ensuring that the most accurate and current information is always applied to benchmarks.
- Data Quality and Availability: Expertly curated, rich data that extends back for decades capture sponsor-agnostic interventional, industry-led FDA track trials. This provides an unbiased view of past success rates from a broad range of pharmaceutical sponsors for comprehensive historical clinical benchmarking data.
- Data Aggregation: Advanced data aggregation methods enable deeper insights and a better understanding of the different factors that impact drug development success (e.g., skipped phases or dual phases in non-standard drug development). Looking at the data from both a path-by-path and a phase-by-phase perspective provides insight into an entire therapeutic area that traditional methods lack.
- Search and Filtering: Advanced and flexible search and filtering options (based on our proprietary ontologies), such as modality, MoA, disease severity, line of treatment, adjuvant status, biomarker, and population characteristics, allow customized deep dives into the data, generating insights even in uncommon or complex treatment settings.
- Improved Methodology: Refined benchmarking methodologies account for different possible development paths without assuming a typical progression. These approaches yield more accurate POS assessments than traditional benchmarking methodologies calculations can.
Approaches drawing on expertly curated clinical and biological data paired with novel methodologies herald a new area of accurate benchmarking in the pharmaceutical industry. If you want to learn how to take your benchmarking to the next level, let’s talk.
*For a phase 2 combo therapy in cancer.