By: Angeliki Lykoudi and Dorita Metallinou, Business Development Associates
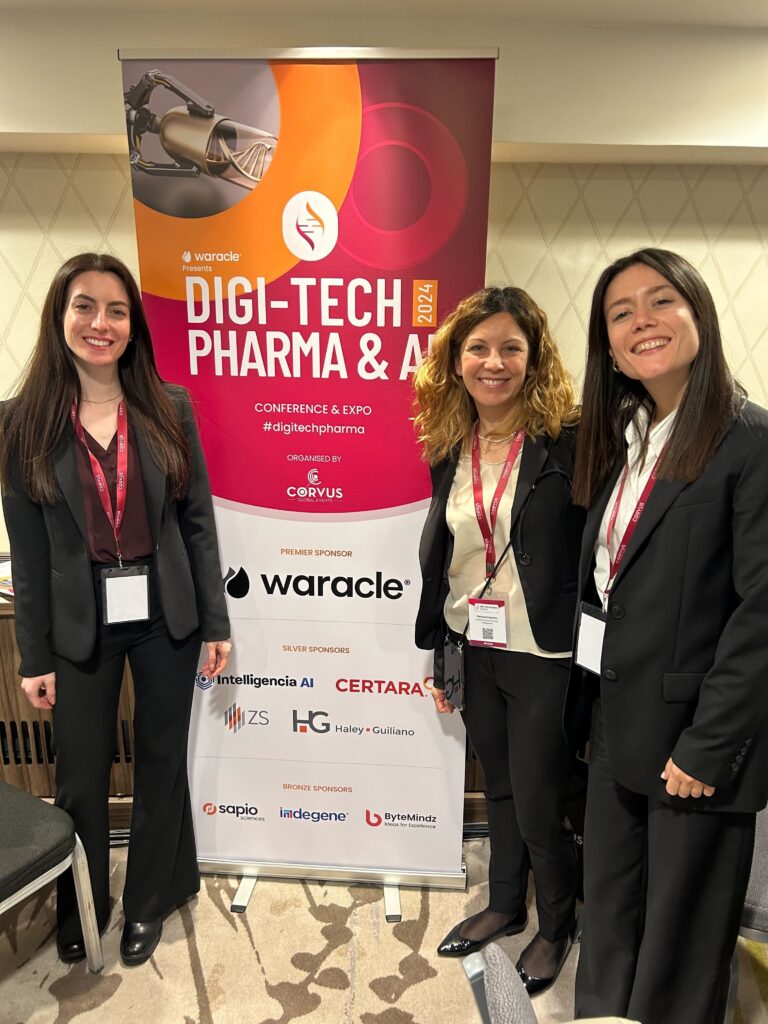
Intelligencia AI’s business development team had the opportunity to attend and sponsor the recent Corvus Digi-Tech Pharma & AI Summit in London. It was an engaging few days with global pharmaceutical leaders sharing the latest from their organizations as well as discussing broader industry developments and news. Here, we summarize our five key takeaways from this important AI and life science conference.
- AI Advances in Drug Discovery
Developing a new drug is a labor-intensive process that requires many lengthy steps, from target identification to clinical trials. Artificial intelligence (AI) holds the potential to expedite this process, lowering both time and costs and facilitating the creation of more effective and efficient treatments. AI can be used at various steps in the drug discovery process, including target identification and drug formulation. Speakers representing big pharmaceutical companies mentioned that they use AI in the drug discovery process to minimize the time it takes for a drug to become available to patients.
Disease modeling and target identification are two critical initial steps in drug discovery that significantly impact the success of drug development and can benefit from AI. AI, specifically deep learning algorithms, can rapidly and accurately analyze vast datasets, such as genomic, proteomic and clinical data, to identify potential targets. It can integrate multi-omics data by building networks that simulate disease pathways or map information from literature to link target diseases with bioactive compounds. This leads to faster target identification and, ultimately, faster access to therapies for patients.
Drug formulation involves determining the proper composition and chemical structure of a new drug. AI algorithms can predict the solubility and stability of compounds, making the formulation process more efficient.
The main challenges of using AI in early drug discovery include the lack of interpretability and inconsistent validation of data across databases. The lack of interpretability refers to the difficulty of understanding how AI algorithms, especially deep learning (DL) models, make decisions; DL models can seem like a black box. This opacity makes it difficult for researchers to trust that the AI’s findings are scientifically valid and reliable. In addition, the quality of AI predictions relies heavily on the data used for training and validation. However, data from different sources can vary widely in terms of quality, format and completeness, leading to AI models that may not be reproducible or generalizable across different datasets. However, in recent years, several AI-developed drugs have entered clinical trials, marking the beginning of a new era in AI-driven drug discovery.
2. Precision Medicine Gets More Precise
Precision medicine is experiencing rapid growth and constitutes a new treatment paradigm. Precision medicine allows healthcare providers to make decisions based on an individual’s unique characteristics rather than basing treatment decisions on data derived from the average patient. It facilitates personalized care for each patient by considering individual genetic background, phenotype, epigenetics, clinical history and lifestyle. Thus, it opens up otherwise unrealizable possibilities in areas such as disease diagnosis, risk prediction, prognosis and treatment response due to differences in environment, physiology and other characteristics.
Pharmaceutical companies have increased their efforts to implement AI to advance precision medicine. AI’s advanced computation and inference capabilities allow these models to reason and learn, thereby improving clinical decision-making.
AI, especially ML algorithms, is mainly applied to therapy planning and risk prediction. Determining the correct treatment for a patient and assessing possible associated risks is one of the most complex challenges in healthcare. AI can facilitate these processes by identifying phenotypes of patients with less common responses to treatment, enabling future genotype-guided treatment.
Despite the significant promise of AI and precision medicine, substantial work remains to be done to test, validate, and transform treatment practices. Researchers face challenges such as obtaining sufficient high-quality data for training algorithms and addressing regulatory and socio-cultural requirements.
3. Drug Repositioning Will Continue to Expand
Due to its ability to save time and cost, drug repurposing, i.e., using an existing drug or drug candidate for a new therapeutic purpose, has garnered significant attention from governments and the pharmaceutical industry.
Interestingly, drug repurposing has emerged as the most utilized approach for identifying therapeutic drugs or potential drug combinations since the COVID-19 pandemic due to the need to identify existing drugs or drug candidates that could help curb the pandemic.
Drug repurposing can benefit from AI because it can quickly detect relationships among various biological entities, such as genes, proteins, diseases, and drugs, helping researchers identify possible new therapeutic areas of application.
Methods such as data mining, machine learning, deep learning and network analysis have been extensively explored with the goal of systematically repurposing drugs, aiming to address the limitations faced by traditional statistical approaches.
In addition, traditional methods often struggle to identify drugs’ molecular targets accurately, especially when working with small datasets from diverse platforms and environmental settings. This can lead to potential inaccuracies in reported findings.
4. AI Will Drive Digital Health Forward
Digital health, or digital healthcare, is a broad term that includes concepts from an intersection between technology and healthcare. Digital healthcare applies digital transformation to the health sciences field, incorporating software, hardware and services. It includes mobile health applications, wearable devices, electronic health records and medical records, telehealth and telemedicine, and precision medicine. Stakeholders in the digital health field include patients, practitioners, researchers, application developers, and medical device manufacturers and distributors. Digital healthcare plays an increasingly important role in today’s healthcare.
There are several applications for AI in digital healthcare. Importantly, AI-enabled digital therapeutics and personalized recommendations are expected to empower consumers to prevent health issues proactively. AI-generated insights will guide diagnosis and treatment decisions, resulting in safer and more effective therapies. Furthermore, intelligent manufacturing and supply chain solutions will ensure the timely delivery of the right treatments and interventions to patients.
Several challenges hinder the advancement of digital health, including the absence of evidence-based standards, privacy concerns, data governance issues, and ethical dilemmas. A significant challenge is the sensitivity of digitized health data, which can lead to privacy concerns. Governments also struggle with managing and protecting data. Ethical issues, such as user consent, arise because many users may not fully comprehend the terms of use when they agree. Moreover, there is insufficient evidence regarding the impact of digital health strategies on health outcomes, cost-effectiveness and system efficiency. Users’ income and socioeconomic status may also influence the effectiveness of telehealth platforms. Specific groups, including minorities, the elderly, and those in low-income or rural areas, may face difficulties understanding and using digital health solutions due to lower levels of health literacy.
5. FAIR Data Is a Must-Have
FAIR data adheres to and stands for the principles of findability, accessibility, interoperability and reusability.
- Findability means data should have unique and persistent identifiers to facilitate sorting and database integration.
- Accessibility ensures that once users locate the data, they understand how to access it, including any necessary authentication and authorization.
- Interoperability involves the integration of data with other datasets.
- Lastly, reusability aims to optimize data reuse by ensuring metadata and data are well-described for replication and combination in various settings.
Implementing FAIR principles enhances both external sharing and internal organizational use.
Applying the FAIR principles in the pharmaceutical industry offers numerous benefits:
- accelerated innovation due to the availability of extensive data sources,
- shorter drug discovery timelines thanks to easy data accessibility,
- elimination of data silos to enhance both internal and external collaboration, and
- improved utilization of advanced analytical methods, including artificial intelligence.
FAIR data is a machine learning enabler as it improves AI model performance by integrating diverse datasets, thereby increasing data points for training. It also enhances data access within pharma/biotech companies, reducing silos and improving data management and governance.
These five takeaways only scratch the surface of the Insights and learnings we brought back from this recent AI and digital-focused conference. As an organization, we had the opportunity to join a panel discussion and share perspectives on how AI is not just a tool for research but is reshaping business models, supports clinical development through improved risk assessment and leads to collaborations with tech companies, the rise of AI-driven biotech firms, and changes in investment strategies.